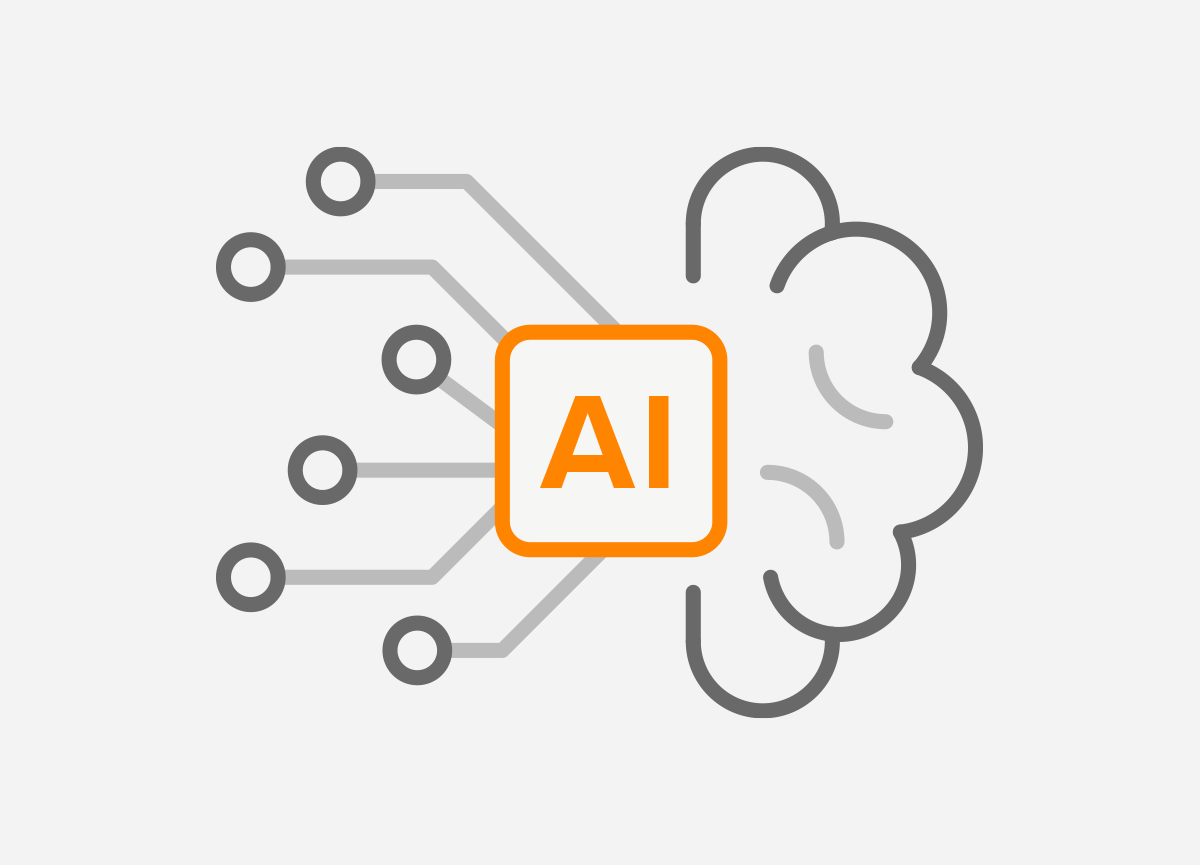
In recent times, the ideas of synthetic intelligence (AI) and machine studying (ML) have gone to the forefront of many industries via the tutorial circle. Specifically, the world of networking has begun to seek out methods to make use of these applied sciences for extra environment friendly administration and operations. This promise is charming: networks that deal with themselves, count on failures earlier than they’ve, enhance visitors on the fly, and modify the buildings with out human intervention. Nonetheless, the fact of AI and ML deployment for community administration is much from straightforward. Many organizations have discovered that complexity, information wants and integration challenges quickly remove the extent of ranges that usually encompass these applied sciences.
On this put up, we are going to discover sensible considerations behind to make the most of AI and ML in community operations, evaluate a bit of historic context and focus on current efforts to create clever community administration options – We will even point out how the platform, such because the Nighting Clever Routing Platform, has approached the issue of community correction – highlighting the remainder of the obstacles by providing stable advantages. Lastly, whereas AI and ML promise, the journey of computerized, self -tinging networks is to alleviate warnings, commerce relations and rising measures fairly than leaping.
Take a short look: from guide connection to computerized intelligence
The thought of clever community administration presents at this time’s machine studying increase for many years. The preliminary efforts of computerized connection instruments have been greater than 20 years, with the platform that has tried to map the dependence of utility to the fundamental community infrastructure. Subsequently, information pulling usually depends on a community layer for SNMP, resembling protocols, and extra proprietary mechanisms for utility information. The aim of those instruments is to grasp the results of the errors and the results of the failure of particular infrastructure components. Nonetheless, this logic was based totally on governance, which was hardly retained by engineering groups, which needed to manually encode each relationship.
The largest drawback was – and nonetheless – that the networks will not be steady. When these units are added, they alter completely, workload shifts, safety insurance policies updates and cloud platforms unfold. Then, in case your connection engine wants to grasp {that a} specific net utility operates on a specific server, behind a specific load steadiness hooked up to a specific firewall, you all this data guide As you must feed. Every week later, a brand new route modified the move of utility, and your connection was outdated. The promise of “clever” communication was quickly indignant with the fact of sustaining a correct, creating mannequin of the community. In apply, these techniques usually lower, or a lot human labor requires that their worth proposal was questioned.
Right this moment, AI and ML are positioned as a remedy for these limits. As a substitute of static, human specified communication, machine studying fashions (in concept) can study community habits samples, detect irregularities, and consider advanced dependence on massive portions of telemetry. Nonetheless, there are basic disadvantages: If you don’t eat AI top quality, correct and complete information, its manufacturing will likely be flawed. AI isn’t a magician who transforms poor information into dependable insights. It routinely routinely detects samples – good or unhealthy – the info given to him.
Getting information in management: AI success base
One of many first obstacles to implementing AI-driving community administration is information hygiene. Community manufactures huge telemetry: routing tables, firewalls, load baller statistics, server matrix, delay measurement and extra. Enterprises can save all this information within the information leak, consider that it’s all the time higher to have “extra information”. But when this determine isn’t taken to regular, mutually up to date and completely up to date, it turns into an unknown swamp fairly than a lake of perception.
Even earlier than serious about “clever” actions, organizations have to ensure they know their infrastructure. The place are the units deployed? Which firmware model are working? Which Overlines Map is influenced? Who’s accountable for sustaining them? With out a fundamental understanding of stable stock, everlasting names, and the atmosphere, the AI mannequin will wrestle. They will make suggestions which can be primarily based on incomplete or stale data, which results in mistrust and reluctance from community engineers.
As well as, for the reason that community is quickly spreading on -love information facilities, a number of public cloudy areas, and containerized microscopes, it isn’t a trivial activity to feed all this complexity into the machine studying mannequin. Primary relationships turn out to be extra sophisticated with each layer of abstraction. If you cannot get a deal with on the “floor reality” of your atmosphere, your ML -powered connective engine will shut the eyes and guess how the items are match collectively.
Gradual and rising progress: to take a seat, crawl, then stroll
Regardless of the hype, most organizations are glorious, within the early phases of AI and ML in community administration. A practical path contains small, further enchancment fairly than a sudden full sovereignty leap.
– Simple observations and warnings:
Initially, machine studying fashions can do some fundamental however nonetheless helpful work: evaluation of efficiency measurement to detect instability. For instance, they will learn to use the “regular” CPU on the router and appear to be directors when used outdoors the anticipated limits. Or they will discover that when a hyperlink delay begins turning upwards, at some instances, pointing to a specific crowd drawback. The detection of such fundamental irregularities will increase the worth and reduces the burden on human operators, which in any other case must be crammed via non -eliminated graphs.
– Occasions and Dependent Relationships:
The marginally extra trendy transfer might embody connecting one occasion to a different. For instance, if a specific swap fails, the system can estimate which purposes have an effect on the map of the system and firewall classes related to this system. Nonetheless, it’s troublesome to make these communication sturdy and dependable. With out precautions and ongoing changes, AI can produce quite a lot of fallacious positives or lose crucial results. Engineers then spend time confirming AI’s output, generally asking whether or not the trouble is price it.
– Planning Predict Upkeep and Capability Plan:
For the reason that machine studying mannequin improves and engineers achieve confidence, they will begin sustaining predictions, resembling predictions when the optical transceiver can fail on the premise of its working temperature and historic age information. This use is a comparatively low danger and infrequently positive aspects actual advantages. Enchancment in capability plan is one other space the place the ML can shine, serving to organizations know the place so as to add bandout or improve units.
-The bond loop automation (any time sooner or later):
Holi Grill-completely computerized, closed loop remedy is much away. The imaginative and prescient the place the AI engine not solely detects an issue but in addition creates community components with out human intervention. Nonetheless, some individuals are prepared to shut their eyes with such duties, trusting within the worry of clashes failures. Not solely technical virtues however cultural modifications are wanted to realize this degree of belief.
The very fact of the hype vs AI/ML in networking
A typical false impression is that AI and ML instruments in networking will shortly displace engineers, or not less than free them from duties. The reality is extra essential. Though some duties could also be offloaded, AI-driving options usually require extra clear effort: Information cleansing, development of infrastructure maps, “atypical” habits description, and community change Continuously updating fashions.
It’s also price noting that AI isn’t incomprehensible. Fashions could make errors, particularly in the event that they must face the scenes they’ve by no means seen earlier than. Skilled fashions on historic information could fail to undertake when new forms of visitors, protocols, or buildings emerge. Missions like networking may be criticized in a field-where closures and fallacious configurations can have extreme consequences-network operators are cautious. They need a confirmed monitor report earlier than turning the keys of the dominion.
As well as, the complexity of recent infrastructure-spreading over the clouds, counting on overalls resembling SD van, and including faux containers-this implies that AI’s work is just used to make use of CPUs Discovering is much extra sophisticated than. Detailed meant networking, the place engineers clarify the specified outcomes and let the system detect the fundamental setting, is theoretically charming. Nonetheless, gaining the best of intention and making certain the ML mannequin can take the best steps to satisfy it. The extra essence of the atmosphere, the extra it’s, it’s straightforward for the AI mannequin to attract fallacious conclusions.
Cultural and organizational challenges
Adopting AI and ML instruments for community administration is not only a technical drawback. It’s also a cultural. Community engineers are educated and examined in educated techniques. They count on orders and reactions, trigger and impact. Machine studying, quite the opposite, is probably going. It really works in prospects fairly than assurances, and its “reasoning” is commonly confused.
This blurry can remove confidence. If an AI platform suggests bettering effectivity, suggesting to remove some flows or modify the firewall guidelines, the engineers need to know what’s the cause. The choice made by the deep studying mannequin may be troublesome to elucidate. To beat this barrier, transparency, sturdy take a look at atmosphere, and insurance policies that guarantee human surveillance.
As well as, community groups, utility groups, and safety groups must cooperate. AI-driving options usually insights from a number of domain-episode efficiency information, safety occasion logs, and infrastructure telemetry and require buy from totally different teams. The misunderstanding or mistrust between these stakeholders can cease AI measures. Organizational preparation and investing in cross -team communication is commonly as essential because the know-how itself.
Quantity IRP, synthetic intelligence and machine studying
Some platforms already present the sensible worth of information -powered resolution -making in community administration and are inspecting methods to make the most of AI and ML sooner or later. Such an answer is our clever rooting platform (IRP), which screens essential matrix-latitude, packet harm, thrupot, and historic reliability to dynamically modify the routing in multi-sophisticated BGP atmosphere. By analyzing telemetry information, IRP easy operations, reduces guide root correction, and releases engineers to deal with extra strategic challenges.
Though the present method to the IRP depends on analytics and algorithmic resolution -making, the Naqsh is contemplating that AI and ML’s superior methods can additional improve its capabilities. Within the close to future, machine studying fashions might help predict routing points earlier than they transfer, enhance the routes extra actively, and assist new methods to cut back danger. In actual fact, the signal within the early of the Q1 2025 plans to introduce the identification of irregularities within the IRP danger discount module, which might allow computerized identification of deviation from atypical visitors samples.
Past the hype
AI and ML are able to altering community administration, however the journey is much from straight improve. Automation’s historic efforts have struggled with the present and correct maping of infrastructure, and faces related challenges in at this time’s AI-driven level of view-although with extra refined instruments –
Lastly, the most effective view relies on realism and warning. AI and ML community can improve the capabilities of engineers, however they don’t change the necessity for talent, curiosity and cautious verification. Sooner or later, a hybrid mannequin will likely be introduced, the place clever instruments assist fairly than increase human choices. Organizations that succeed are those who spend money on the proper information, the best course of and the best tradition – accepting that the “fully impartial and clever” networks are a marathon, not a marathon.